Associate Professor
Decision Sciences and MIS
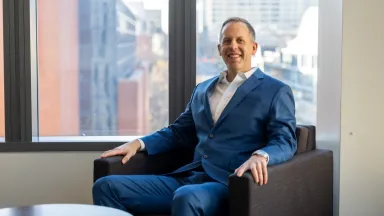
Gerri C. LeBow Hall 623
Personal Website LinkedIn Google Scholar SSRNBiography
Dr. Schneider’s research enables organizations to create useful data while protecting consumer data privacy. He creates flexible, but careful statistical methodologies that preserve the patterns required for profitable business decisions. Outside of privacy, he focuses on forecasting revenue and sales using new data.
By translating the 5% of usable theory to practice, Dr. Schneider served as a scientific lead for the largest FinTech, pharma, insurance, and retail companies. He has been used for pre-IPO validation of data science and forecasting models in FinTech. He also specialized in improving analytics and anonymization frameworks at numerous Fortune 500 companies.
His research has been published in the Harvard Business Review, Journal of the Royal Statistical Society, Marketing Science, International Journal of Research in Marketing, Journal of Consumer Psychology, International Journal of Forecasting, and Journal of Privacy and Confidentiality. Dr. Schneider also teaches Data Privacy Strategy to corporate executives through eCornell.
Dr. Schneider holds a PhD in Statistics and prior to Drexel, he was an Assistant Professor of Marketing at Northwestern University and a Visiting Scholar at the Samuel C. Johnson Graduate School of Management at Cornell University. He was also the Director of Research at Fort Rock Asset Management LLC, a fund of hedge funds based in Portland, Oregon. He holds a PhD and MS in Statistics from Cornell University, a MS in Public Policy and Management from Carnegie Mellon University, and a BS in Quantitative Economics from the United States Naval Academy. Before finishing his PhD, he was employed at the RAND Corporation from 2008 to 2013 and served in the U.S. Navy as an Officer of the Deck and Surface Warfare Officer on the USS Boxer from 2003 to 2005.
Areas of Expertise
- Data Privacy
- Time Series Forecasting
Selected Works
Articles
Lee, Jinwook, and Schneider, Matthew J., Geometric series representation for robust bounds of exponential smoothing difference between protected and confidential data. Annals of Operations Research (Sep 2023).
Li, Shaobo, Schneider, Matthew J., Yu, Yan, and Gupta, Sachin, Reidentification Risk in Panel Data: Protecting for k-Anonymity. Information Systems Research (Year 2022).
Gupta, Sachin, Moutafis, Panos, and Schneider, Matthew J., To Protect Consumer Data, Don’t Do Everything on the Cloud. Harvard Business Review (Digital Article) (Jun 2021).
Schneider, Matthew J., Protecting Survey Data on a Consumer Level. Journal of Marketing Analytics (Year 2020).
Gupta, Sachin, and Schneider, Matthew J., Protecting Customers’ Privacy Requires More than Anonymizing Their Data. Harvard Business Review (Digital Article) (Jun 2018).
Schneider, Matthew J., Jagpal, Sharan, Gupta, Sachin, Li, Shaobo, and Yu, Yan, A Flexible Method for Protecting Marketing Data: An Application to Point-of-Sale Data. Marketing Science (Year 2018).
Schneider, Matthew J., Jagpal, Sharan, Gupta, Sachin, Li, Shaobo, and Yu, Yan, Protecting customer privacy when marketing with second-party data. International Journal of Research in Marketing 34 (Year 2017):593-603.
Schneider, Matthew J., and Gupta, Sachin, Forecasting sales of new and existing products using consumer reviews: A random projections approach. International Journal of Forecasting 32 (Year 2016):243-256.
Schneider, Matthew J., and Gorr, Wilpen, ROC-based model estimation for forecasting large changes in demand. International Journal of Forecasting 31 (Year 2015):253-262.
Schneider, Matthew J., and Abowd, John, A new method for protecting interrelated time series with Bayesian prior distributions and synthetic data. Journal of the Royal Statistical Society 178 (Year 2015):963-975.
Iacobucci, Dawn, Posavac, Stephen, Kardes, Frank, and Schneider, Matthew J., Toward a more nuanced understanding of the statistical properties of a median split. Journal of Consumer Psychology 25 (Year 2015):652-665.
Abowd, John, Schneider, Matthew J., and Vilhuber, Lars, Differential Privacy Applications to Bayesian and Linear Mixed Model Estimation. Journal of Privacy and Confidentiality 5 (Year 2013).